Imagine a researcher trying to understand the health outcomes of a diverse population. They can’t just survey everyone – that would be time-consuming and potentially costly. Instead, they might decide to divide the population into smaller groups based on factors like age, gender, or socioeconomic status. These groups, known as strata, would then be sampled proportionally to reflect the overall population. This is precisely what stratification is all about – dividing a population into subgroups to ensure accurate and representative sampling.

Image: www.dochub.com
Stratification is a powerful statistical technique with wide-ranging applications. It allows researchers to analyze data more effectively, draw more accurate conclusions, and make better decisions. In this article, we’ll delve into the intricacies of stratification, exploring various real-world examples, and understanding how it enhances research and decision-making processes.
Understanding Stratification
What is Stratification?
Stratification is a sampling technique used in research to divide a population into smaller subgroups, or strata, based on shared characteristics. This technique aims to ensure that each stratum is adequately represented in the sample, leading to more accurate and representative findings.
The primary goal of stratification is to reduce sampling error. By dividing the population into homogenous groups, researchers can minimize the variability within each stratum, making it easier to draw conclusions about the entire population.
Why is Stratification Important?
Stratification is crucial for several reasons:
- Enhanced Accuracy: Stratification helps researchers gather more accurate data as it ensures that each subgroup is properly represented in the sample.
- Reduced Sampling Error: By minimizing variability within each stratum, stratification reduces sampling error, leading to more reliable findings.
- Increased Representativeness: Stratification enhances the representativeness of the sample, making it more likely to reflect the characteristics of the overall population.
- Targeted Analysis: Stratification allows for focused analysis of specific subgroups, providing valuable insights into the variations within the population.
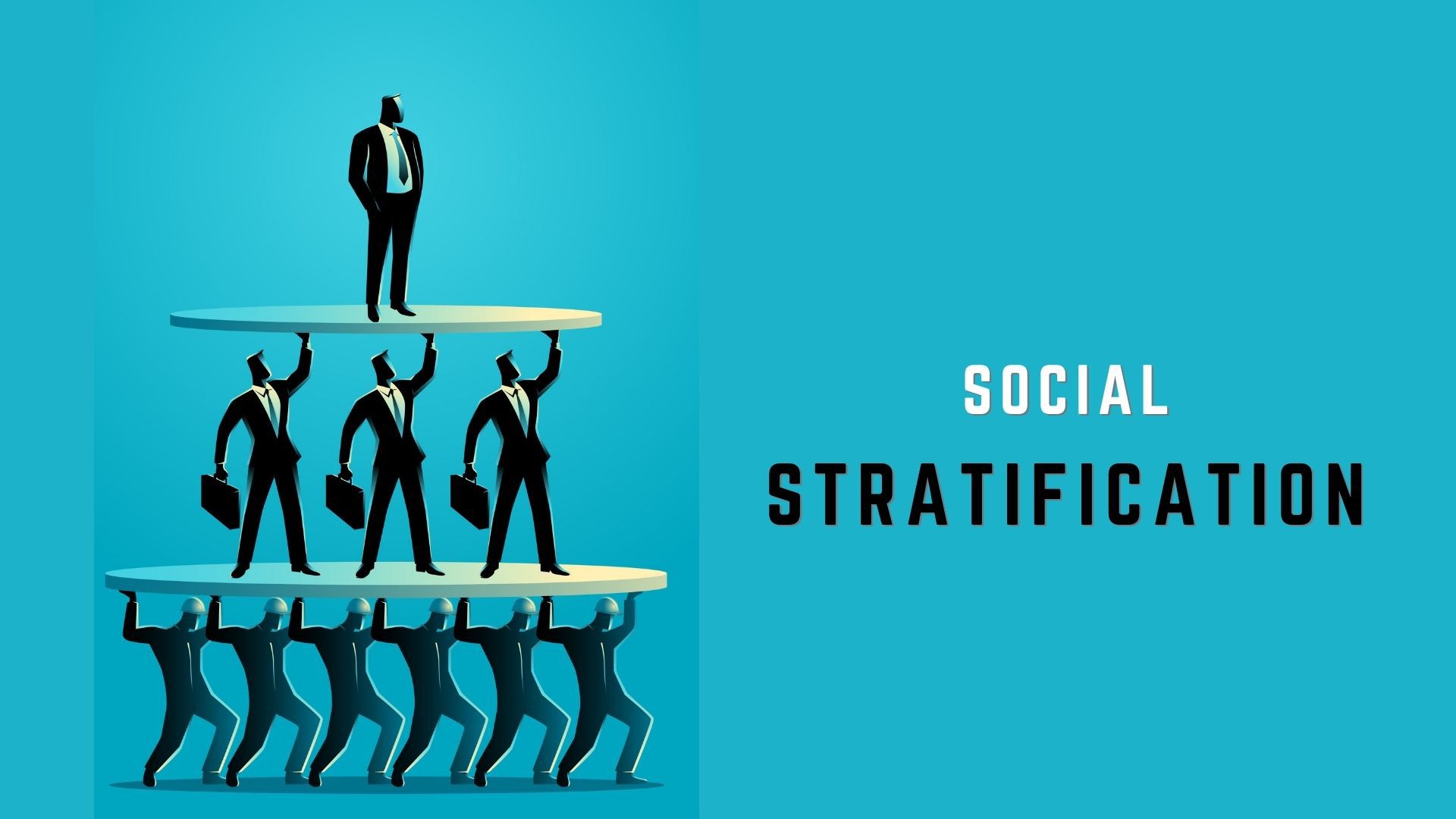
Image: complianceportal.american.edu
Real-World Stratification Examples
Stratification is applied in diverse fields, including market research, public health, social science, and political polling. Here are some compelling examples:
1. Market Research
Imagine a company launching a new product targeted at young adults. To understand their preferences, they might stratify their target audience based on age, gender, and income level. This ensures that the sample adequately reflects the demographics of their target market, leading to more accurate insights and informed marketing strategies.
2. Public Health
Stratification is crucial for studying the prevalence of diseases. For instance, researchers might stratify a population based on geographic location, socioeconomic status, and access to healthcare. This allows them to analyze disease patterns and identify potential risk factors within specific subgroups, ultimately informing public health interventions.
3. Political Polling
Political pollsters frequently use stratification to ensure their samples accurately reflect the political landscape. They divide the electorate into strata based on factors such as age, gender, race, and political affiliation. This approach enables them to predict election outcomes with greater precision and analyze voting trends among different demographics.
4. Educational Research
Stratification helps educational researchers understand variations in student performance. For example, they might stratify students based on socioeconomic background, academic ability, or learning styles. This allows them to identify specific needs and develop targeted educational programs that cater to different student groups.
Latest Trends and Developments
With advancements in technology and data analysis, stratification continues to evolve. Here are some emerging trends:
- Big Data and Stratification: As organizations collect vast amounts of data, stratification is becoming increasingly crucial for analyzing these complex datasets and extracting meaningful insights.
- Machine Learning and Stratification: Machine learning algorithms are being integrated into stratification techniques to automate the process of identifying suitable subgroups and optimizing sample selection.
- Dynamic Stratification: Researchers are developing dynamic stratification approaches that allow for real-time adjustments to strata based on changing population demographics and data patterns.
Expert Tips and Advice
Here are some practical tips for employing stratification effectively in your research:
- Identify Relevant Stratification Variables: Carefully select variables that significantly influence the outcome you are studying. Consider factors such as age, gender, location, socioeconomic status, and specific characteristics relevant to your research.
- Ensure Strata are Homogeneous: Aim for strata that are relatively homogenous within themselves, meaning that individuals within each stratum share similar characteristics. This helps minimize variability and improve analysis accuracy.
- Maintain Proportionality: Ensure that the size of each stratum in the sample is proportional to its size in the overall population. This ensures that your findings accurately represent the population as a whole.
- Consider Stratification for Analysis: Even if you don’t stratify your sampling, consider stratification during data analysis to explore variations among different subgroups and gain deeper insights.
By following these tips, you can effectively apply stratification to enhance the quality and reliability of your research findings.
Frequently Asked Questions
Q: When is stratification most appropriate to use?
A: Stratification is most effective when dealing with heterogeneous populations where certain subgroups exhibit significant variations in the variable under study. It’s also recommended when you want to ensure accurate representation of specific subgroups within the sample.
Q: What are some limitations of stratification?
A: One limitation is identifying the most relevant stratification variables. Incorrect choices can lead to less effective stratification. Additionally, if you have too many strata, it can become complex and challenging to analyze the data.
Q: How do I decide on the number of strata to create?
A: The number of strata depends on the specific research objectives, population size, and availability of data. Generally, aim for a balance between having enough strata for meaningful analysis while keeping the complexity manageable.
Stratification Examples
Conclusion
Stratification is a powerful statistical technique that empowers researchers to draw more accurate and comprehensive conclusions from their data. By consciously dividing populations into subgroups and ensuring their proportional representation in the sample, researchers can improve the reliability of their findings and make better informed decisions.
Are you interested in discovering more examples of stratification in action? Share your thoughts in the comments below! Let’s discuss how this technique can be applied in your own research or decision-making process.